서론
본 포스트에서는 Computer vision 기반의 3가지 얼굴 인식 및 탐지 방법을 연구합니다.
- eigenfaces (1부)
- Local Binary Patterns Histograms (2부)
- Fisherfaces
1. Eigenfaces face recognition
EigenFaces face recognition은 PCA 방법(Principal Component Analysis) 방법을 사용하여 얼굴 데이터를 high demention에서 low demention으로 처리하고 얼굴 데이터의 주요 구성 정보를 얻은 다음 얼굴 인식을 완료한다.
EigenFaces 얼굴의 인식 단계
- create the EigenFace recognizer
- train the model with a known image
- recognizer to identify the unknown image and confirm its identity
위의 얼굴 인식 단계의 설명은 code level로 설명을 하였습니다.
PCA(Principal Component Analysis)
PCA는 분포된 데이터들의 주성분을 찾는 방법, 보통 여러차원의 큰 데이터를 3차원, 2차원으로 낮추어 보기위해 사용된다.
여러차원에서 데이터가 보여졌을 지라도 데이터가 실제로 있는 차원은 더 낮은 차원일 수 있다. 그래서 PCA를 사용하여 데이터가 실제로 존재하는 차원을 찾는 것이라고 할 수 있다.
PCA는 데이터 하나 하나에 대한 성분을 분석하는 것이 아니라, 여러 데이터들이 모여 하나의 분포를 이룰 때 이 분포의 주 성분을 분석한다.
주성분 이란 데이터들의 분산이 가장 큰 방향 벡터를 의미 한다.
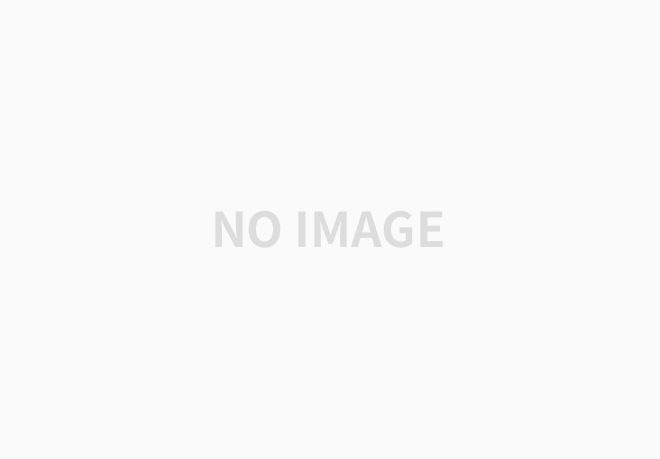
Code(Python)
import cv2
if __name__ == "__main__":
train_images = list()
labels = list()
test_image = None
recoginer = cv2.face.EigenFaceRecognizer_create()
recoginer.train(train_images, labels)
label, confidence = recoginer.predict(test_image)
print("Matching label :", label)
print("Credibility: ", confidence)
대략 위의 코드를 참고해서 직접 실습을 해보면 된다.
인식기를 생성한 후, 학습을 진행한다. 그리고 학습된 인식기를 이용해 테스트 이미지를 예측하고 label과 정확도를 출력하는 코드이다.
참조
[OpenCV-Python]33.OpenCV face detection and recognition -- face recognition
[OpenCV-Python]33.OpenCV face detection and recognition -- face recognition Posted by saadlive on Wed, 05 Jan 2022 22:41:50 +0100 33.OpenCV face detection and recognition - face recognition preface face recognition is to further judge a person's identi
programmer.ink
https://darkpgmr.tistory.com/110
[선형대수학 #6] 주성분분석(PCA)의 이해와 활용
주성분 분석, 영어로는 PCA(Principal Component Analysis). 주성분 분석(PCA)은 사람들에게 비교적 널리 알려져 있는 방법으로서, 다른 블로그, 카페 등에 이와 관련된 소개글 또한 굉장히 많다. 그래도 기
darkpgmr.tistory.com
'Computer Vision' 카테고리의 다른 글
Computer vision based Skin Detector (0) | 2022.07.20 |
---|---|
LBP (Local Binary Pattern) for Face Recognition (0) | 2022.07.18 |